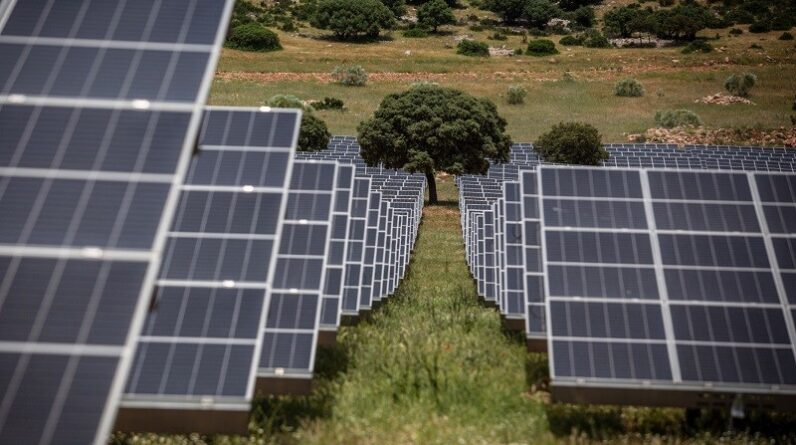
[ad_1]
This article was written by Don Huff, Global Head of Client Services and Operations, Michael Tam, Supply Chain Data Product Manager, Maris Serzans, Supply Chain Data Science at Bloomberg, alongside collaborators at Databricks. You can read the original article here.
Supply chain resiliency is becoming increasingly important to corporations. Procurement managers must maintain transparent oversight across the entire supply chain network and be aware of potential risks. Supply chains in recent years have faced a litany of disruptions, culminating in one stoked by a global pandemic that kept workers at home and paralyzed supply chains around the world. As companies emerge from the pandemic, leaders are asking themselves and their teams how to prepare for the next big disruption.
According to a 2022 McKinsey and Company report surveying the practices of manufacturing companies, two-thirds of companies implemented new supply chain risk management practices over the past 12 months. Among such initiatives include implementing processes to monitor supplier-related risks, which is particularly important for companies that prioritize uninterrupted, on-time customer delivery. Companies are also experiencing shorter decision-making cycles, given a recent surge in supply chain volatility.
Corporations need end-to-end visibility and automation across their supply chain network, which calls for a comprehensive data solution. Bloomberg delivers supply chain data for over 100,000 companies with historical data stretching back to 2006, providing corporations with strong visibility into their supply chains, several supplier tiers deep. In this blog, we will explore Bloomberg’s risk data spanning ESG, sanctions, and default risk data to help customers surface potential concerns in a company’s supply chain.
Case Study: Analyzing supply chain risk at a leading auto manufacturer
We focus on the supply chain network of a global auto manufacturer, transforming complex datasets in order to extract actionable insights. We highlight three risk factors our clients consider when assessing their supply chain risks: default risk, sanctions risk, and climate risk.
1. Default risk
Bloomberg’s proprietary default risk model uses inputs such as a company’s debt to calculate default probability. By combining supply chain and default risk data, we can identify upstream suppliers which are financially distressed. Within this automaker’s supply chain, we identified eleven suppliers with a one-year probability of default of over 10%, which can be considered distressed. A supplier facing liquidity issues may trigger disruptions in the flow of goods/components and consequent delivery challenges for the customer.
2. Sanctions risk
Similar to default risk, we can use entity data from DL+ tables and easily identify sanctioned entities in the global auto manufacturer’s supply chain network. While only one direct supplier (tier 1) is sanctioned, multiple tier 2 and tier 3 suppliers have sanctions applied to them.
According to McKinsey and Company, only 10% of supply chain leaders surveyed by the consulting firm in 2022 had visibility into their tier-3 suppliers. Using Bloomberg’s supply chain and sanctions data, customers can identify indirect exposure to sanctioned companies and engage with their suppliers to identify alternatives.
3. Climate risk
Corporations are increasingly monitoring their Scope 3 emissions – those generated from supply chain activities. Here, we demonstrate how mutual users can easily surface entity-level emissions data over several tiers of the automaker’s suppliers via DL+. Although the global automaker itself does not have high emissions intensity, there are several suppliers across all tiers whose carbon intensity metrics are lagging behind peers. The automaker should determine whether they are comfortable with those suppliers’ practices or they want to proactively modify their supply chain.
Supply chain research workflows are rapidly transforming with the advent of sophisticated cloud platforms. It has allowed corporations to harness data in powerful ways, particularly in areas such as supply chain analytics where traceability and visibility are critical. The ability to combine DL+ datasets in a unified analytics environment can accelerate insights about the state of your business and competition.
Our case study highlights three examples in which Bloomberg data can be used to surface actionable insights. It is possible to productionalize such risk models into scheduled workflows shared across your organization. There is still much to explore – for instance, our team is working on integrating climate risk data to surface companies’ exposure to environmental threats across the supply chain.
To learn more about Bloomberg’s data click here.
[ad_2]
Source link