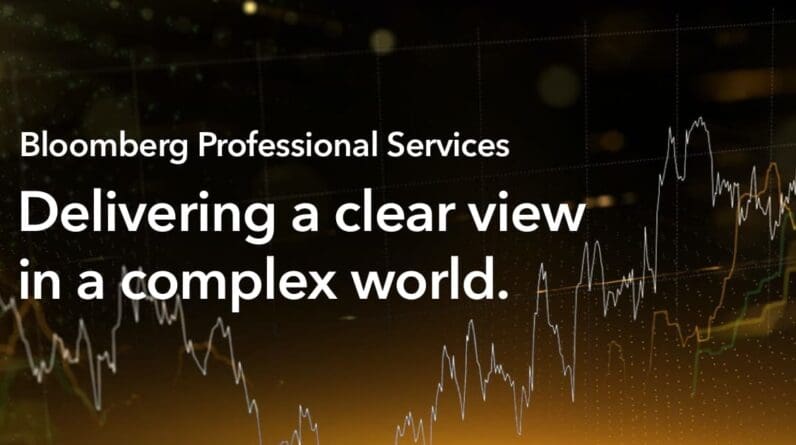
[ad_1]
This article was written by Ashlee Vance. It appeared first on the Bloomberg Terminal.
A new startup called MatX from former Google engineers reflects a renewed enthusiasm for chipmakers.
It’s taken about 25 years, but Silicon Valley finally feels like the old-school Silicon Valley again.
Nvidia Corp. has so totally dominated the market for the chips that power artificial intelligence software that other companies have decided they’re willing to pursue the often-disastrous exercise of designing their own semiconductors. History tells us that designing a chip from scratch takes years, costs hundreds of millions of dollars and usually ends without victory. Yet the promise of AI is simply so great that people have decided they must try.
Two of these brave souls are Mike Gunter and Reiner Pope. They’ve founded a company, MatX, whose objective is to design silicon specifically for processing the data needed to fuel large language models. In case you’ve been hiding in a bunker for the past year and a half, LLMs are the basis for things such as OpenAI Inc.’s ChatGPT and Google’s Gemini, and they require an obscene amount of very expensive chips to run. If a company were able to make cheaper, faster, AI-friendly chips, it would be poised to do very well in a world of ever-expanding AI software.
Gunter and Pope previously worked at Alphabet Inc.’s Google, where Gunter focused on designing hardware, including chips, to run AI software, and Pope wrote the AI software itself. Google has for years been building its own AI chips called tensor processing units. These chips, though, were first designed before LLMs really started to take off and are too generic for the current task at hand, according to the MatX executives. “We were trying to make large language models run faster at Google and made some progress, but it was kind of hard,” Pope says, speaking publicly about his company for the first time. “Inside of Google, there were lots of people who wanted changes to the chips for all sorts of things, and it was difficult to focus just on LLMs. We chose to leave for that reason.”
Nvidia’s dominance in the AI silicon market is something of an accident. The company started out making chips known as graphics processing units (GPUs) to speed up video games and certain computer design jobs. The Nvidia chips excel at handling lots and lots of small tasks, and it just so happened that they ran the AI software that started taking off about a decade ago much better than other types of chips made by Intel Corp.
Nvidia splits up the real estate on its GPUs to handle a wide variety of computing jobs, including moving data around the chip. Some of its design choices cater more to past eras of computing than to the AI boom and come with performance tradeoffs. The MatX founders contend that this extra real estate adds unneeded cost and complexity in the new era of AI. It’s taking a clean-slate approach, designing silicon with one large processing core aimed at the single purpose of multiplying numbers together as quickly as possible—the central task at the heart of LLMs. The company is betting—and it’s an all-or-nothing type of bet—that its chips will be at least 10 times better at training LLMs and delivering their results than Nvidia’s GPUs. “Nvidia is a really strong product and clearly the right product for most companies,” Pope says. “But we think we can do a lot better.”
MatX has raised $25 million, with its most recent funding round being led by the AI investing duo of Nat Friedman and Daniel Gross. The company is located in Mountain View, California, a couple of miles away from Silicon Valley’s first transistor factory, Shockley Semiconductor Laboratory, and has dozens of employees beavering away on the chip that the company plans to have in hand next year. “The MatX founders are symbolic of a trend in our AI world,” Gross says, because they’re “taking some of the best ideas developed at some of the largest companies, which are a little bit too slow-moving and too bureaucratic, and commercializing them on their own.”
If AI software continues on its current path, it will create a huge need for costly computing. The models under development are estimated to cost about $1 billion apiece to train, and their successors are expected to cost $10 billion to train. MatX predicts it can have a booming business by winning over a number of the major AI players including OpenAI and Anthropic PBC. “The economics of these companies are completely backwards from typical companies,” Gunter says. “They’re spending all this money on computing, not on salaries. If things don’t change, they will run out of money.”
Silicon Valley, as the name makes clear, used to be awash in chip companies. There were dozens of startups, and even computing giants Hewlett-Packard, IBM and Sun Microsystems all made their own chips. In more recent history, however, Intel quashed many of these efforts through its dominance of the PC and server markets, while companies such as Samsung Electronics Co. and Qualcomm Inc. came to dominate smartphone components. Because of these trends, investors began to move away from committing capital in chip startups, seeing them as much more costly, time-consuming and risky than software. “Around 2014, I would visit venture capital firms, and they’d removed all their partners who knew about semiconductors,” says Rajiv Khemani, a chip expert who invested in MatX. “I would be staring at people who had no clue what I was saying.”
The rise of AI, however, has changed the risk-versus-reward equation. Companies with vast resources—Amazon.com, Google and Microsoft among them—have invested in designing their own tensor chips for AI jobs. And several years ago, startups such as Groq Inc. and Cerebras Systems Inc. appeared on the scene with a first surge of AI-specific chips. But these products were designed before major technical AI breakthroughs led to the rise of LLMs as the dominant story in AI. The startups have had to adjust to the sudden interest in LLMs and try to tweak their products on the fly. MatX likely represents the beginning of yet another surge of chip startups focusing on LLMs from the ground up.
The huge problem with entering the chip industry is that it takes three to five years to design and manufacture a new chip. (Nvidia, of course, won’t be standing still during this time and, in fact, announced a much faster version of its GPUs this month.) Startups have to predict where technology trends and the competition will be, with little room for errors that could slow down production. Software companies usually have to rewrite their code to run on new semiconductors, a costly and time-consuming process that they’ll do only if they expect a large payoff for making the switch. The rule of thumb is that a new chip must be at least 10 times better than what has come before it to have any chance of persuading customers to shift all their code.
For his part, Gross predicts that we are in the early stage of building out the infrastructure needed to support the shift to AI as the dominant form of computing. “I think we are moving into a semiconductor cycle that will make the others seem pale by comparison,” he says. If he’s right, then there are almost certainly new chip empires yet to be created.
[ad_2]
Source link